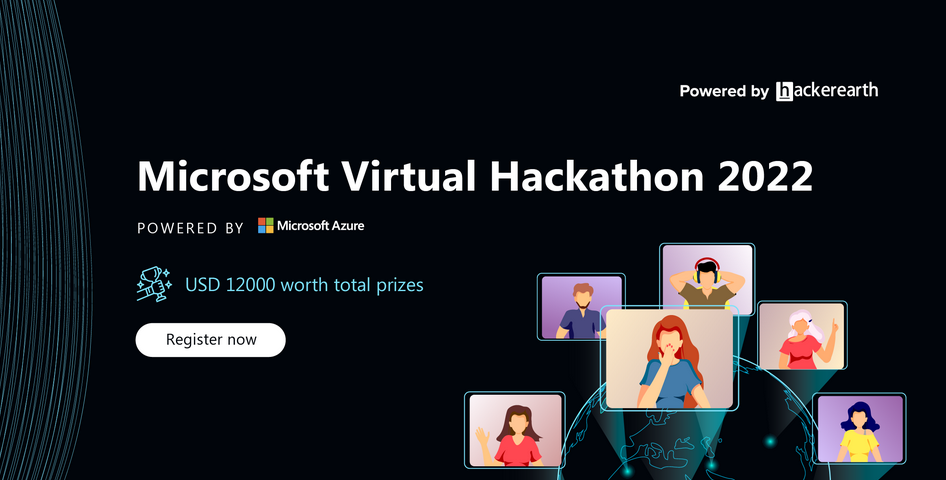
Data Literacy – Business Intelligence
Data Literacy
As self-service analytics is getting traction, it is essential to have the ability to write, read, interpret, and understand data across the enterprise.
Individual knowledge workers should have the ability to understand the analytics regarding business value or outcome. Data and analytics are pervasive in all domains of business. Data literacy is fundamental for self-service analytics and today’s data-driven culture. One must be able to identify, access, integrate, and manage both internal and external data and draw insights relevant to business use cases.
Some of the ways to increase data literacy are as follows:
• Create organization-wide standards, terminology, business glossary,data dictionary, and dataflow diagrams. You can use industry-wide data models.
• Increase data visualization skills and increase business curiosity about data by using trainings and certification.
• Regularly measure the overall adoption of BI initiatives.
• Increase the initiative to address cultural and data literacy challenges within strategies and programs.
Edge analytics: Edge analytics is a subpart of analytics where processing is executed in distributed devices, gateways, or servers located in the edge (where data is created and executed).
Figure 7-6 demonstrates the flow of data from source, processing, and output flow for edge analytics.
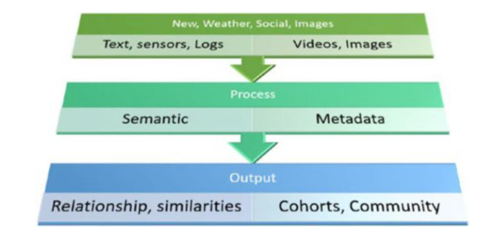
Figure 7-6. Edge analytics dataflow
Growth in 5G networks, combined with mobile edge computing and the connectivity and demand for IOT, is increasing the scale, complexity, and number of use cases. Given the demand for real-time event analytics and decision-making, autonomous and fault-tolerant applications will eventually likely increase across industries.
Some edge analytics benefits are faster response time, reduced network bottlenecks, better data filtering, and reliability.
Governance issues related to sensitive or regulated data, and the storage location of that data, which can violate sovereign regulations, combined with mobile edge computing are some of the challenges that can constrain D&A (data management and analytics) teams.
Real-time intelligence: Real-time analytics are integrated in business operations to process current and contextual data and prescriptive actions with which to respond. It provides automation in decision-making and support. This can be achieved by a combination of machine learning, event stream processing, augmented analytics, data quality, and the business rules engine.
It is important to have the capability to trigger an automated response by sending messages to other systems that can trigger business processes.
The first level of D&A capabilities is descriptive analytics, and the second level is predictive modeling. The third level is prescriptive analytics. This means using analytics for supplying prescriptive information about the best available action in the current context.
The growing complexity—and the desired scalability, speed, and automation of decision-making—fuel the adoption of decision intelligence. Constant monitoring of systems looks for threats and opportunities, and offers suggested or automated responses to those events.
Advanced intelligent systems require the following:
Full integration of real-time analytics with cross-functional business processes New, modern skills like enterprise architect, advanced data engineering, and data scientist, along with cross-functional advance knowledge of business required to implement such a system
Here are some of the examples of real-time BI use cases:
Providing real-time observations, using sensors, about drivers’ behavior, creating a safety score insurance companies can use to recommend products; find the purchasing behavior of insurance products; and sale and marketing decisions for insurance companies.
Another example is providing GPS/positional data tracking of vessels to dry bulk operators, which can collect operational and engine data that can impact business decisions regarding reduction of fuel consumption and operational cost. Being transparent and proactive in sharing such data and supply-chain metrics with customers can improve the business and save consumption and operation costs. These benefits can traverse all domain areas, functions, and layers of a business.
Shorter latency of data means proactive decisions can be made by identifying additional business capabilities and use cases from active and passive metadata. While the first step is to collect data to monitor operations for the purpose of observation, there are a few actions/decisions that can be taken instantly, and there are other decisions that need due diligence. Decisions that require due diligence need shared data kept in data store for later.
Archives
- August 2024
- July 2024
- June 2024
- May 2024
- April 2024
- March 2024
- February 2024
- January 2024
- December 2023
- October 2023
- September 2023
- August 2023
- July 2023
- May 2023
- March 2023
- January 2023
- December 2022
- November 2022
- August 2022
- June 2022
- April 2022
- March 2022
- January 2022
- December 2021
- October 2021
- August 2021
- June 2021
- April 2021
- March 2021
- January 2021
Leave a Reply